Pharmacovigilance in the Age of AI: Enhancing Drug Safety with Technology
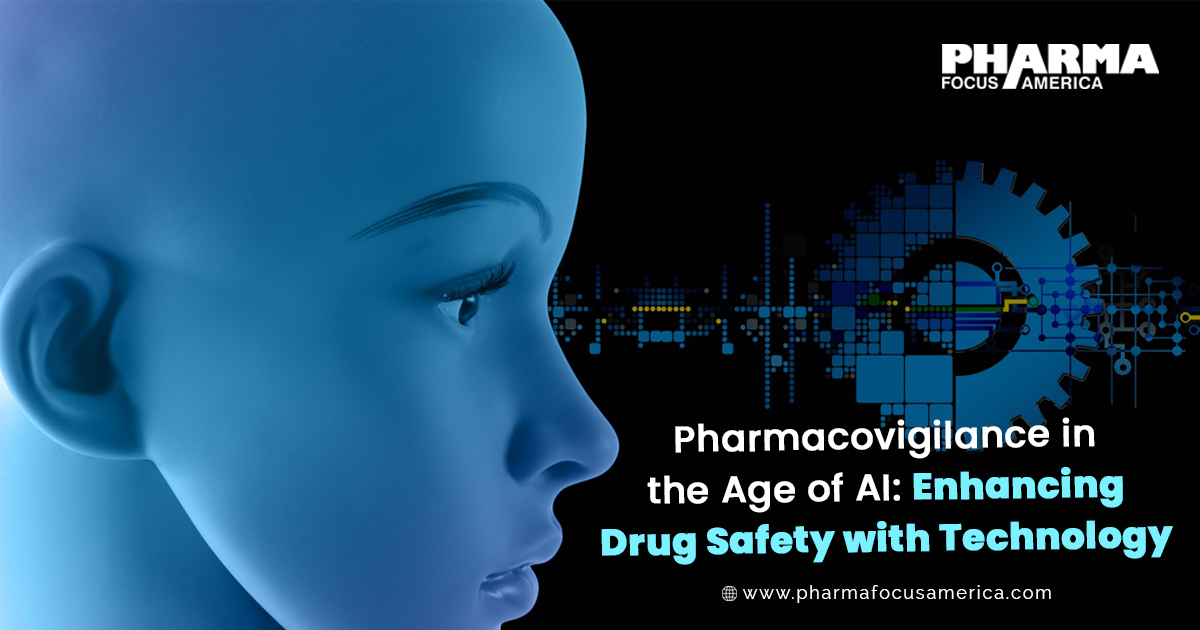
AI is revolutionizing pharmacovigilance by enhancing drug safety through advanced technologies like machine learning and natural language processing. These innovations enable efficient, accurate detection of adverse drug reactions, timely interventions, and comprehensive data analysis. Despite challenges, AI-driven pharmacovigilance promises improved patient outcomes and proactive risk management in healthcare.
Introduction:
Pharmacovigilance, the science of detecting, assessing, understanding, and preventing adverse effects or any other drug-related problems, is a critical component of healthcare. With the advent of artificial intelligence (AI), the landscape of pharmacovigilance is rapidly evolving. AI offers unparalleled opportunities to enhance drug safety, streamline processes, and provide more accurate and timely insights into drug-related risks. This article delves into the transformative role of AI in pharmacovigilance, exploring its benefits, challenges, key technologies, and future trends.
The Role of AI in Pharmacovigilance
AI technologies, including machine learning (ML), natural language processing (NLP), and data mining, are revolutionizing pharmacovigilance by enabling more efficient and accurate detection of adverse drug reactions (ADRs). These technologies can analyze vast amounts of data from various sources, including electronic health records (EHRs), social media, clinical trials, and spontaneous reporting systems, to identify patterns and signals that might indicate potential drug safety issues.
Key Technologies in AI-Driven Pharmacovigilance
1. Machine Learning (ML): ML algorithms can learn from historical data to predict future adverse events. By training models on large datasets, ML can identify subtle patterns and correlations that might be missed by traditional methods. This predictive capability is crucial for proactive pharmacovigilance, allowing for early intervention and mitigation of potential risks.
2. Natural Language Processing (NLP): NLP enables the extraction and analysis of unstructured data from various text sources such as medical literature, social media posts, and patient reports. By understanding and interpreting human language, NLP can identify mentions of ADRs and contextual information that might be relevant for pharmacovigilance.
3. Data Mining and Signal Detection: Advanced data mining techniques can sift through large datasets to detect signals of ADRs. These techniques can identify associations between drugs and adverse events, even when the events are rare or the signals are weak. Signal detection algorithms can prioritize these signals for further investigation by pharmacovigilance professionals.
Benefits of AI in Pharmacovigilance
Enhanced Efficiency: can automate many routine tasks in pharmacovigilance, such as case processing, data entry, and report generation. This automation reduces the workload on human resources, allowing them to focus on more complex and value-added activities.
Improved Accuracy: AI algorithms can process and analyze data with high accuracy, reducing the risk of human error. This precision ensures that potential safety signals are not overlooked and that false positives are minimized.
Timely Detection of ADRs - AI enables real-time monitoring and analysis of data, leading to the timely detection of ADRs. Early detection allows for quicker responses, which can prevent widespread harm and improve patient safety.
Comprehensive Data Analysis - AI can integrate and analyze data from diverse sources, providing a more holistic view of drug safety. This comprehensive analysis can uncover insights that might be missed when data is considered in isolation.
Discover more: https://www.pharmafocusamerica.com/articles/ai-enhances-drug-safety-in-pharmacovigilance
- Art
- Causes
- Crafts
- Dance
- Drinks
- Film
- Fitness
- Food
- Juegos
- Gardening
- Health
- Home
- Literature
- Music
- Networking
- Other
- Party
- Religion
- Shopping
- Sports
- Theater
- Wellness